Orientation: There is startlingly little economic research on the South African wildlife sector which contributes toward disputes regarding the economic contribution of the sector.Research purpose: The purpose of this article is to put into context the relative economic contribution of the wildlife ranching sector, as opposed to other land-use options in South Africa. Motivation for the study: Growth in the wildlife ranching sector at the cost of other traditional farming practices resulted in disagreements amongst various role players about the impact thereof on the national economy. The controversy can most probably be explained by different beliefs, coupled with the lack of a proper understanding and quantification of the wildlife ranching sector’s contribution toward the economy. Research methodology: The study employed a Social Accounting Matrix-based Leontief multiplier analysis for South Africa. Main findings: Results from the multiplier analysis revealed that developments within the wildlife ranching sector are likely to make a relatively more superior contribution towards the economy, especially when compared to similar land-use options such as extensive livestock production. Practical/managerial implications: It has been acknowledged by both academia and private sector that a major need exists for more research on the South African wildlife ranching industry, specifically looking at issues such as the industries, economic and social contributions, potentials and constraints. The research, therefore, contributes toward the depth of economic information and research regarding the South African wildlife sector. Contribution/value added: The research provides valuable information in dealing with the ‘popular belief’, especially amongst some of South Africa’s decision makers, namely, that growth in the wildlife ranching sector is not or does not have the ability to contribute significantly toward economic and socioeconomic factors.
The South African wildlife ranching sector has experienced tremendous growth and development; from what was seen as being just an alternative method of production on marginal land to a multidimensional industry. Bothma (2002), as cited by Cousins, Sadler and Evans (2010), defines wildlife ranching as the management of free-living animals on large, primarily fenced areas on private or communal land that are utilised for the purpose of hunting, live-game sales, trophy hunting, wildlife meat or tourism. The same definition will be adopted for the purposes of the study.It is widely considered that the insertion of a monetary value on wildlife has been critical in motivating the initial revolution, contributing toward the remarkable growth experienced in the wildlife ranching sector since 1991 (Chardonnet et al. 2002; Lindsey, Roulet & Romañach 2007). Today, this sector is globally recognised, resting on several consumable and non-consumable pillars (i.e., recreational hunting, trophy or biltong hunting, venison, live-game trade and ecotourism), with hunting and ecotourism being the main contributors toward the economy of the sector (Cloete, Taljaard & Grové 2007). Despite the growth and transformation experienced within the sector, several discussions remain about the contribution of the wildlife ranching sector toward, or the impact thereof on, the local economy. At a political level, it is frequently being argued that the growth in the wildlife ranching sector has taken so-called productive land out of the system, inflicting higher levels of poverty. To the contrary, however, role players within the sector believe that the transition to wildlife ranching has had a positive impact, namely, contributing toward overall economic growth, creating a number of skilled and semi-skilled employment opportunities, as well as furnishing a source of foreign exchange, with these benefits spilling over to various recipients and sectors. These views are supported by the studies of Chardonnet et al. (2002), Van der Merwe, Saayman and Krugell (2007), Musengezi (2010), Booth (2010), Saayman, Van der Merwe and Rossouw (2011a; 2011b), and Child et al. (2012), who revealed that the wildlife sector has the potential to contribute significantly toward economic growth and development. For example, results from a case study by Musengezi (2010), which included eight properties near the Kruger National Park, reveal that the financial returns of wildlife properties exceed those of extensive commercial beef production and provide a significant number of employment opportunities. Analogous research by Van der Merwe et al. (2007) and Saayman et al. (2011a; 2011b) not only discloses the significant contribution of the hunting industry toward real Gross Domestic Product (GDP) and employment, it also reveals noteworthy spill-over effects toward the rest of the economy. Moreover, PriceWaterhouse (1994), as well as Taylor (2002) and Langholz and Kerley (2006), as cited by Child et al. (2012), conclude that wildlife enterprises not only create employment opportunities, but also more specialised employment opportunities, which increase wage bills some 20- to 32-fold. Nonetheless, these findings have not encumbered any of the controversies around the economic impact of the wildlife ranching sector. The reason for the continued controversy may well be explained by the fact that the contribution of the wildlife ranching sector, as presented by the aforementioned studies, was not in a comparable framework. Although comparisons were drawn in the study by Musengezi (2010), it does not enable the reader to grasp the total economic benefits resulting from wildlife ranching compared with all other comparable land use options. Therefore, unless a proper understanding of the economic contribution of the South African wildlife ranching sector compared with other similar land use options is established, the controversy may remain. With the aforementioned in mind, the study attempts to quantify the potential economic contribution of the wildlife ranching sector compared with other land-use options and, specifically, traditional livestock production. However, the official economic size of the wildlife ranching sector in South Africa is unknown. Therefore, results and comparisons will be reported on a Rand-to-Rand basis, that is, potential contribution for every Rand’s worth of production in the respective sectors considered. This approach will not provide absolute values; however, it will shed light on whether growth in the wildlife ranching sector at the cost of other traditional farming practices is of economic benefit or not. The following section provides a brief literature review on different approaches for measuring the economic contribution of the wildlife sector, followed by a detailed discussion on the methodological approach, data used and results and, finally, concluding remarks.
Different ways of measuring the contribution of the wildlife ranching sector
|
|
Before reviewing different ways of measuring the economic contribution of the wildlife ranching sector, it is worth clarifying the concept. Ritchie and Goeldner (1994), as cited by Saayman et al. (2011a), argue that economic impact can be defined as ‘the net change in a host community that results from [the] spending in a given area’. Weisbrod and Weisbrod (1997) suggest that the economic contribution of a specific activity or sector be viewed as ‘the effects on the level of economic activity’ and can be measured according to the (i) business output, (ii) value added or Gross Domestic Product (GDP), (iii) employment and (iv) wealth or aggregate personal income.According to Tisdell and Wilson (2004) and Tisdell (2006), different approaches can be followed to determine the economic contribution of the wildlife ranching sector. These include welfare economics or economic impact analysis. The first approach, namely, welfare economics, involves social cost–benefit analysis. This approach implies that the economic worth or value of wildlife conserved or consumed as a result of a specific programme should be compared to the cost of the programme. If the net benefits are positive, this indicates that the programme has economic merit and gains more worth the larger the net economic benefits become (Tisdell 2007; Weisbrod & Weisbrod 1997). Tisdell (2007) elaborates by arguing that the most common method used by economists to determine the worth or value of wildlife is by estimating ‘the maximum amount of money individuals would be willing to pay for the continuing presence [or consumption] of the wildlife’. Therefore, following the welfare economics approach, economists try to estimate the total economic value of wildlife, which includes the use and non-use values of wildlife (Tisdell 2007). The second method, namely, economic impact analysis, gauges the economic importance of wildlife through its impact on factors such as the ones highlighted by Weisbrod and Weisbrod (1997). Tisdell (2007) reports that economic impact analysis has a more limited scope than a social cost–benefit analysis, which estimates total economic value. One reason for this is that social cost–benefit analysis takes into account non-marketed components, for example, existence value, whereas economic impact analysis only takes into account the marketed (or commercial) economic components associated with wildlife (Tisdell 2007). However, Dorfman (1993) stresses that the results from a social cost–benefit analysis approach are less objective and more controversial than those from an economic impact analysis. Moreover, Tisdell (2007) highlights the fact that it is both challenging and costly to estimate the economic value or worth of wildlife. Thus, given the scope of the study, the economic contribution of the wildlife ranching sector and other alternative land-use options will be measured according to the factors deemed appropriate by Weisbrod and Weisbrod (1997).
Research method and design
|
|
Model selection
Jafri and Buland (2006) report that the most popularly-used impact models include Input-Output (IO)- and Social Accounting Matrix (SAM)-based models. Additionally, Townsend (1997) argued that the measurement of backward and forward linkages have become standard practice in IO-type analysis. Taljaard (2007) elaborated by suggesting that when developments in the analysis of transactions within an economy are expanded to include the entire economy and its linkages, the original IO transaction matrix can be set in the wider accounting framework of a SAM. A SAM-based model can thus be seen as a better instrument for quantifying the contribution of the wildlife industry toward the selected economic and socioeconomic aggregates.According to Round (2003), the SAM is not a model, but rather a depiction of a set of macro-micro data for a specific economy. If designed suitably and reinforced by survey data and other evidence, it does propose some important and useful features about the socioeconomic character of and the association between the structure of production and the distribution of income in an economy. McDonald and Kirsten (1999) emphasised that the importance of SAMs as a basis for wide-ranging economic models has increased substantially over time. These models (which use SAMs as a database) normally comprise computable general equilibrium (CGE), partial equilibrium and multiplier models. CGE models are regarded as the dominant framework amongst these models. Dixon et al. (1992) are of the same view, suggesting that CGE models are preferred to partial equilibrium models. This is because the production and consumption sides of the economy in question are determined concurrently in a CGE model framework. However, CGE models are generally used to measure ex-ante the possible impact of a set of policy changes, which are modelled by means of exogenous shocks (Bellù 2011). Therefore, when considering the aim of the study (i.e., to determine the contribution of the wildlife ranching sector toward selected economic and socioeconomic aggregates), a CGE model will not be appropriate. On the other hand, a simpler fixed-price model (such as a SAM multiplier model) will provide a better indication of the contribution of the wildlife ranching sector in South Africa.
Social Accounting Matrix Leontief multipliers
A SAM is a disaggregated, comprehensive and consistent representation of the flow of all economic transactions taking place within an economy. In addition, ‘it attempts to classify various institutions to their socioeconomic backgrounds instead of their economic or functional activities’ (Chowdhury & Kirkpatrick 1994). Accordingly, the SAM multiplier model links income and household consumption, thereby permitting an appraisal of the full effects of specific changes to the economy (Golan et al. 2000).According to Golan et al. (2000), total output equals total demand in a SAM, as is shown in Equation 1. [Eqn 1]
where z denotes a vector of total output, Az equals the sum of endogenous demands and x represents exogenous demands. The shares matrix (A) (also known as the technical coefficient matrix) represents value added, endogenous production and household expenditures as shares of total expenditure. The exogenous accounts are typically government, the capital account and domestic and foreign trade. By solving Equation 1, it is possible to determine the impact of a change or shock in exogenous demand on total output, accounting for all variations in endogenous demand which result from exogenous change. This can be done by altering Equation 1 as follows: [Eqn 2]
where , [Eqn 3]
to facilitate: [Eqn 4]
The M matrix (also known as the multiplier matrix or Leontief inverse) is able to capture the impact that an exogenous change in demand has on value added, endogenous production and household expenditures. Moreover, it reflects the fact that an increase in demand for the output from a particular sector (e.g. that of the wildlife ranching sector) creates a further demand for intermediate goods produced by other firms. The knock-on effect of this is that these other firms then pay their workers higher wages to produce these goods and the workers, as consumers, spend their extra income on goods and services. Consequently, in equilibrium, the vector sums up for all firms, factors and households in the economy the direct effects resulting from the shock itself as well as the indirect effects in the form of new wage payments, household expenditures and producer supply feedbacks. ‘More formally, each sectoral multiplier ( ) represents the induced income flow to account i account j, as a result of one unit of exogenous expenditure placed on sector (Golan et al. 2000). ‘If the change in exogenous demand (whether from investment demand, a government policy, or export demand) is for goods, the multiplier is a production multiplier. If the exogenous flow is directed to a household, the multiplier is an income transfer multiplier’ (Golan et al. 2000). In addition to output and in order to measure the contribution of the wildlife ranching sector, the GDP or value added, income and employment multipliers are calculated from the initial expenditure, using average value added/output, household income/output and employment/output coefficients respectively. When applying the above method, it is also important to understand the underlying assumptions. Accordingly, Pyatt (1988) explains that a SAM multiplier model shows how an economy operates, given a set of assumptions which should be interpreted within the appropriate context. There are three main assumptions underlying the SAM multiplier framework that have the effect of weakening its general applicability. According to Taljaard (2007), two of the three assumptions include, firstly, fixed relative prices and, secondly, perfect elastic supply conditions, that is, excess production capacity in all sectors. The implication of the latter is that the SAM multiplier model understates the impact of an increase in household income on the demand for luxury goods, whilst at the same time overstating the impact on demand for necessities, whereas the former implies that only quantities adjust to clear markets. Sadoulet and De Janvry (1995) explain that these assumptions lead to the central (and third) assumption in this type of analysis, namely that sectoral production is completely demand driven and that the underlying production function assumes constant returns to scale and no substitution amongst different inputs. The implication here is that not only can downstream industries maintain the required flow of intermediate goods, but there are always sufficient underutilised resources to meet increases in demand. This also implies that both job gains and losses are treated as permanent and instantaneous. Although these assumptions may prove restrictive in some analyses, they are not particularly problematic for this study. Because the comparisons made are within the same methodological framework, this ensures that the results are, in fact, comparing like with like.
Data used
The article uses the 2006 SAM for South Africa compiled by Conningarth Economists and available from the Development Bank of Southern Africa (DBSA 2009). This specific SAM contains the most detailed disaggregation of South Africa’s primary sector (i.e., deciduous, citrus and subtropical fruit farming; vegetable, livestock, game and dairy [milk only] farming; forestry; fishing; cereal and crop farming; poultry farming; and other agriculture). Moreover, by using a SAM, rather than an IO table, to calculate multipliers it is possible to consider not only output/production multipliers, but also GDP and labour income multipliers, which can expose ‘important distributional effects from external demand-side shocks’ (Breisinger, Thomas & Thurlow 2009).The SAM provides a snapshot of the economy at a point in time. The specific 2006 SAM used consists of 209 accounts, which are classified as either endogenous or exogenous. The endogenous group of accounts include 48 activities, 48 commodities, 48 factors of production and 52 institutions. The exogenous group of accounts include five types of taxes and subsidies, three levels of government, three capital accounts, a savings-investment balance and the rest of the world (ROW). Using the rich detail as captured in the SAM, whilst keeping in mind the aforementioned assumptions of fixed prices and no supply constraints, multiplier analysis can expose the inter-linkages and interactions between the various detailed accounts (Rossouw & Cloete 2014). In other words, multiplier analysis will not only reflect the direct contribution toward set economic and socioeconomic parameters, but will also inform on the indirect and induced contributions.
As mentioned, it is important to bear in mind that the justification of SAM-based economic multipliers as a methodology for impact analysis rests on the appropriate interpretation of the results. Thus, the key assumption of fixed relative prices and perfectly elastic supply of economic multipliers needs to be kept in mind when interpreting the results from the multiplier analysis. Moreover, the data reflects a snapshot of the national economy during 2006 – the multipliers are calculated for 2006-constant South African Rands.The following subsections will report on inter-linkages of the wildlife ranching sector (as compared with other land-use options) with the rest of the economy, the production, GDP/value added, employment and household income multipliers in order to determine the potential contribution of the wildlife ranching sector compared with alternative land-use options.
Inter-linkages in the economy
We first look at the backward and forward multipliers of each of the different agricultural subsectors of the South African economy. The backward multiplier captures the linkages of a sector with the upstream industries (industries from which the sector purchases inputs), whilst the forward multiplier captures the linkages with the downstream industries (industries to whom the sector sells inputs). Thus, ‘forward multipliers capture the changes in the downstream sectors’ production as a result of a one-unit increase in the value added in a sector whilst backward multipliers capture the changes in the upstream industries’ production driven by a one-unit increase in the final demand of a given sector’ (Commission of the European Communities [CEC] 2007).This makes it possible to categorise the different primary sectors in relation to the intensity and nature of their inter-linkages with the rest of the economy. A sector is considered to be forward-oriented if the size of its forward multipliers is greater than the average size of forward multipliers across all sectors of the economy. Similarly, a sector is considered to be backward-oriented if the size of its backward multipliers is greater than the average size of backward multipliers across all sectors of the economy. On this basis, it is possible to classify ‘key sectors’ as those which are simultaneously backward- and forward-oriented, that is, the sectors with the strongest inter-linkages across the whole of the economy. This analysis also allows for distinction between sectors whose inter-linkages are spread over many sectors in the economy versus those concentrated in one or a few sectors (CEC 2007). Box 1 presents such a categorisation of the different agricultural subsectors in the South African economy. Figure 1 shows a graphical classification of the activities in the South African SAM, according to the size of their forward and backward linkages (i.e., based on the classification framework of Box 1). The figure shows that the wildlife ranching sector falls within the ‘weak sector’ categorisation, which is neither backward- nor forward-oriented. Only two sectors, namely poultry farming and dairy farming, are downstream-oriented, that is, they have strong inter-linkages with downstream sectors (captured by the forward multipliers). The remaining alternative land-use sectors also fall within the ‘weak sector’ category.
 |
FIGURE 1: Backward and forward linkages of the primary sectors.
|
|
BOX 1: Backward and forward inter-linkages: Typology of sectors.
|
This basic analysis of the inter-linkages of the wildlife ranching sector can be extended by a comparison of different metrics, such as each sector’s share of total employment, combined with its contribution factor (or multiplier) in terms of production, GDP/value added, employment and household income. From this comparison it becomes clear that some sectors have a large presence in the country, but may not contribute significantly to the generation of spillover effects in the form of indirect or induced jobs. On the other hand, however, some sectors represent only a small portion of the economy but have large contribution factors or multiplier effects.
Production multipliers
Figure 2 summarises the production multipliers (direct, indirect and induced effects) for the respective agricultural sectors as portrayed in the SAM. According to Conningarth Economists (2006), the economic term ‘production’ refers to the total turnover (quantity produced multiplied by the corresponding price) generated by each activity and/or sector in the economy, which can be measured as the sum of the intermediate inputs plus the total value added by the specific sector. This is similar to what Weisbrod and Weisbrod (1997) referred to as business output, namely, ‘includes the full (gross) level of business revenue, which pays for cost and materials as well as generating business income’. Moreover, the direct multiplier for each of these sectors will be equal to 1 (production divided by itself).
The real contribution of production multipliers within the context of the study is the indirect and induced impacts of, especially, the agricultural subsectors that compete for the same natural resources, that is, wildlife ranching versus extensive livestock farming. The indirect multiplier reflects the impact a particular sector will have on all other industries that supply inputs (materials) for the operations taking place in the sector. These ‘backward linkages’ are important, since they measure the broader impact changes the direct sector will have on the economy (Conningarth Economists 2006). The induced effects measure the economic impact which results from salaries and wages paid to employees in both the particular or direct activity and the input-supplying sectors. Figure 2 shows the production multipliers (direct, indirect and induced) for the various agricultural subsectors included in the analysis. The indirect multiplier for the wildlife ranching sector is the sixth highest at 0.82 – only 0.03 lower than that of extensive livestock farming (0.85). This implies that a R1 increase in the production of the wildlife ranching sector has a backward effect of R0.82 (an increase in sales) on the economic sectors supplying inputs to the wildlife ranching sector. The induced effect of the wildlife ranching sector amounts to 1.02 – 0.01 higher than that of the extensive livestock sector (1.01) – indicating that a R1 increase in the production of the wildlife ranching sector will result in a R1.02 increase in salaries and wages paid to employees in both the wildlife and other sectors supplying inputs to the sector. Combined, the total multiplier (2.84) for the wildlife ranching sector is slightly lower than that of the extensive livestock farming sector. Therefore, from a production point of view, it can be argued that the transition from extensive livestock to wildlife will have no significant negative impact on the rest of the economy. On the contrary, when considering the findings of Musengezi (2010), who argues that the wildlife ranching is more profitable than extensive livestock farming (i.e., higher production/income on the same size/piece of land), one can argue that despite having a slightly smaller production multiplier, the wildlife ranching sector is likely to make a larger contribution toward the overall economy when compared with extensive livestock farming. Whilst the presentation in Figure 3 suggests that the ideal economy would contain only those sectors with higher multipliers, this is never possible. It is, however, feasible to identify those sectors (as alternatives for land use) ‘that are good candidates for investment or enhancement. That is, to the extent that demand-side opportunities exist, supply-side investment will result in a stronger economy’ (CEC 2007).
 |
FIGURE 3: Production multipliers vs. percentage labour contribution.
|
|
Relative to other land-use alternatives, the wildlife ranching sector is a driver of economic activity, but with a relatively small contribution to direct job creation. An upswing in production as a result of investment in wildlife ranching generates a larger knock-on effect in terms of production, but generates fewer direct jobs compared with extensive livestock production.
Gross domestic product and/or Value added multipliers
For the purposes of the multiplier analysis, GDP or value added is defined as the sum of the value added over all sectors of the South African economy. This refers to the second factor as alluded to by Weisbrod and Weisbrod (1997) in determining a sector’s economic contribution. The value added multipliers for the different agricultural subsectors are shown in Figure 4. It is important to note that value added multipliers only measure the value added proportion, thereby eliminating the ‘double counting’ effect that typical output multipliers include by adding all additional output for all sectors (that is the input to other sectors). The value added multipliers are, therefore, considerably smaller than plain output multipliers.The estimated direct value added multiplier for the wildlife ranching sector amounts to 0.42, which indicates that R420 000 of direct value is added for every R1 million of primary production in the sector. Moreover, R380 000 of indirect worth will be added for every R1m in primary production. This will result in an additional R500 000 worth of salaries and wages paid to employees involved in either the wildlife ranching sector itself or secondary sectors that process primary products produced on wildlife ranches. Compared to the value added multipliers for the extensive livestock sector, the total multiplier (direct, indirect and induced combined) reveals that production in the wildlife ranching sector is likely to make a slightly larger contribution toward the national economy when considering the same value of production from the extensive livestock farming sector (see Figure 4). In other words, growth within the wildlife ranching sector (i.e., transition of land previously being used for extensive livestock farming to wildlife ranching) will be beneficial to the overall economic growth in South Africa.
 |
FIGURE 4: Gross domestic product/Value added multipliers.
|
|
However, Figure 5 shows that, relative to other land-use alternatives, the wildlife ranching sector has a low GDP/value added multiplier effect, along with a relatively small contribution to direct job creation. An upswing in activity resulting from investment in wildlife ranching generates a relatively smaller knock-on effect in terms of GDP/value added and generates fewer direct jobs. Conversely, a downturn in activity would result in a relatively smaller loss in GDP/value added and less direct jobs being lost.
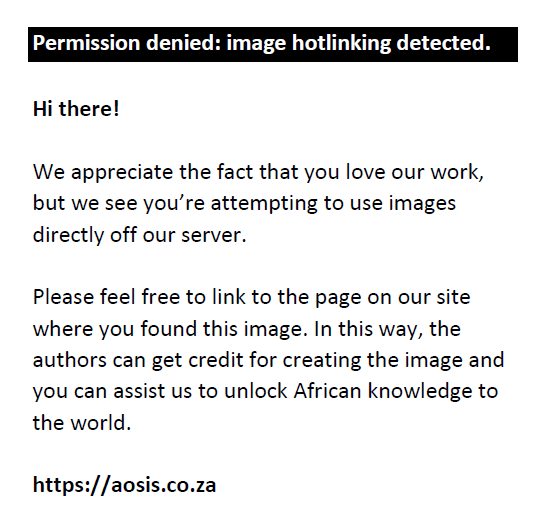 |
FIGURE 5: Gross domestic product/Value added multipliers versus percentage labour contribution.
|
|
Employment multipliers
Weisbrod and Weisbrod (1997) suggest that employment is another factor that needs to be considered when determining the overall economic contribution of a specific sector. Conningarth Economists (2006) explains that employment multipliers measure the employment creation and indicate the extent to which each sector contributes toward the creation of employment opportunities and, ultimately, each sector’s contribution toward distributing salaries and wages amongst the various types of labours. This, in turn, should impact directly on the alleviation of poverty, that is, by the creation of wealth in South Africa.Figure 6 shows the employment multipliers for the various agricultural sectors. The direct employment multiplier for the wildlife ranching sector amounts to 7.54, implying that for every R1 million worth of production the subsector will generate almost eight full-time employment opportunities. In addition, the backward linkages (indirect multiplier) indicate that an additional three full-time employment opportunities will be generated within the sectors/industries supplying inputs to the wildlife ranching sector for every R1m of production. Combined with the induced effects (employment creation because of the additional salaries and wages paid) the wildlife ranching sector will generate an estimated 15 full-time employment opportunities for every R1m worth of production. Compared to other agricultural subsectors, the wildlife ranching sector is ranked seventh highest in terms of its potential to generate employment opportunities per R1m worth of production (see Figure 6). More importantly, it is clear from the employment multiplier analysis that the potential of the wildlife ranching sector to address contentious issues such as poverty alleviation is slightly higher than sectors that compete directly with wildlife for the same agricultural resources.
Figure 7 shows that, relative to other land use alternatives, the wildlife ranching sector has a relatively large employment multiplier effect (thus, a larger indirect and induced employment effect), along with a relatively small contribution toward direct job creation. An upswing in activity resulting from investment in wildlife ranching generates a relatively large knock-on effect in terms of indirect and induced job creation, but generates fewer direct jobs. Conversely, a downturn in activity would result in a relatively greater loss in indirect and induced jobs, but with less direct jobs being lost.
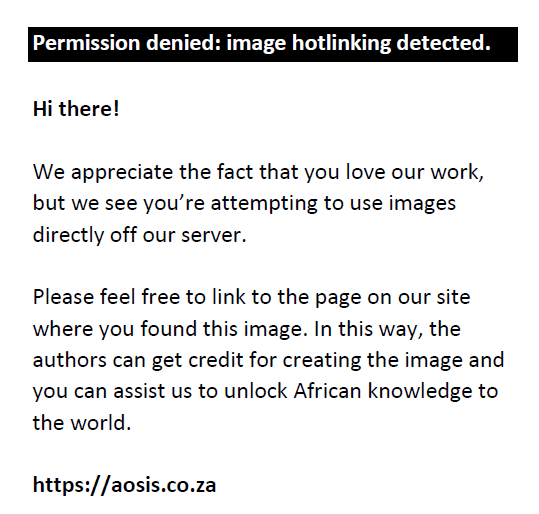 |
FIGURE 7: Employment multipliers versus percentage labour contribution.
|
|
Household income multipliers
The household income multipliers focus mainly on the payments (wages and salaries) destined for South African households from activities within the various sectors and reflect the fourth variable as referred to by Weisbrod and Weisbrod (1997). It is a known fact that most economic hardship resulting from unemployment and extreme poverty exist within the rural low-income households. Therefore, household income multiplier analysis separated households into ‘low income’ and ‘the rest’ in order for it to be meaningful in the South African context (see Figure 8).From Figure 8, it can be seen that the wildlife ranching sector has a household income multiplier of 0.83. This means that South African households working in the wildlife ranching sector will earn, on average, about 83 cents for every R1 of additional demand. Of the 83 cents, an estimated 4 cents will be destined for low-income households. The distribution of income amongst low-income and other households working in the wildlife ranching sector is similar to other agricultural subsectors. This is because of the way in which capital (property, machinery, etc.) is owned and the way in which wages accrue predominantly to the workers. Based on income distribution alone, it can be argued that the potential of the wildlife ranching sector to alleviate poverty is similar to that of other sectors that compete directly for the same agricultural resources, such as extensive livestock farming (see Figure 8). However, when considering the potential for employment creation, it is clear that more households (including low-income households) are likely to benefit from growth in the wildlife sector at the expense of extensive livestock farming.
Figure 9 shows that, relative to other land-use alternatives, the wildlife ranching sector has a low-household income multiplier effect, along with a relatively small contribution toward direct job creation. An upswing in activity resulting from investment in wildlife ranching generates a relatively smaller knock-on effect in terms of household and generates fewer direct jobs. Conversely, a downturn in activity would result in a relatively smaller loss in household income and less direct jobs being lost.
 |
FIGURE 9: Household Income multipliers versus percentage labour contribution.
|
|
Holistically, it is clear from the results that the wildlife ranching sector in South Africa has the potential to make a more meaningful contribution toward economic growth, employment creation and poverty alleviation, especially when compared with other sectors that compete directly with wildlife for the same natural resources. Whilst having a slightly smaller production multiplier compared with extensive livestock, higher profitability per hectare is likely to result in a larger contribution toward the overall economy. The same can be seen in terms of GDP or value added and the employment multiplier effects, with the wildlife ranching sector reporting higher value added and employment multipliers compared with extensive livestock production. In other words, the wildlife ranching sector is likely to make a slightly larger contribution toward the national economy and generate more full-time employment opportunities when considering the same value of production from the extensive livestock farming sector.The inter-linkages of the wildlife ranching sector with the rest of the economy suggest that the sector is neither forward- nor backward-oriented. This means that that neither the forward nor backward multipliers are greater than the average size of forward or backward multipliers across all the economic sectors. However, the results reveal that although the wildlife ranching sector only accounts for a relatively small portion of the economy, it possesses relatively large contributory factors or multiplier effects. This is especially true in terms of the secondary or spillover effects. For instance, the wildlife ranching sectors production multiplier in comparison with the percentage labour absorption reveals that an upswing in production will result in a larger knock-on effect in terms of production whilst at the same time generating fewer direct employment opportunities. Similarly, although having a relatively large employment multiplier, the knock-on effect in terms of indirect and induced employment from an upswing in economic activity will be greater than the direct employment opportunities that are created within the sector itself. These findings, coupled with those from various other studies, contradict the view that a transition of land previously being used for extensive livestock production to wildlife ranching will encumber growth and inflict higher levels of poverty. Although the results of this article provide a more in-depth insight into the contribution of the wildlife ranching sector toward various economic factors; further research is recommended on the entire network of paths through which these structural relationships in an economy lead to the ultimate effects (i.e., contributions as portrayed in the article). The latter will provide valuable information in terms of revealing the network of transmission channels between the wildlife ranching sector and other sectors of the economy – providing an even more holistic view of the contribution made by wildlife ranching toward various other sectors in the national and international economies.
Competing interests
The authors declare that they have no financial or personal relationship(s) which may have inappropriately influenced them in writing this article.
Authors’ contributions
P.C.C. (North-West University) was responsible for the writing of the article and a portion of the analytical work and R.R. (North-West University) was responsible for most of the analytical work in the article.
Bellù, L.G., 2011, ‘Analyzing policy impacts and international price shocks: Alternative Computable General Equilibrium (CGE) models for an aid-dependent less-industrialized country’, viewed 21 July 2014, from
http://www.fao.org/fileadmin/user_upload/tcas/projects/cge_models_apr2011.pdfBothma, J du P., 2002, ‘Some economics of wildlife ranching’, in B.L. Penzhorn (ed.), Proceedings of a Symposium on Game Ranch Planning and Management, 1–2 November 2002, pp. 23–40, The Wildlife Group, South African Veterinary Association, Onderstepoort, South Africa. Booth, V.R., 2010, ‘The contribution of hunting tourism: How significant is this to national economies?’, in a joint publication of FAO and CIC, Contribution of Wildlife to National Economies, FAO and CIC, Budapest. Breisinger, C., Thomas, M. & Thurlow, J., 2009, Social accounting matrices and multiplier analysis: an introduction with exercises, International Food Policy Research Institute, Washington, DC. Chardonnet, P., Des Clers, B., Fischer, J., Gerhold, R., Jori, F. & Lamarque, F., 2002, ‘The value of wildlife’, Revue Scientifique et Technique (International Office of Epizootics) 21(1), 15–51. Child, B.A., Musengezi, J., Parent, G.D. & Child, G.F.T., 2012, ‘The economics and institutional economics of wildlife on private land in Africa’, Pastoralism: Research, Policy and Practice 2(18), 32 pages. Chowdhury, A. & Kirkpatrick, C., 1994, Development policy and planning: An introduction to models and techniques, Routledge, London & New York.
http://dx.doi.org/10.4324/9780203423905 Cloete, P.C., Taljaard, P.R. & Grové, B., 2007, ‘A comparative economic case study of switching from cattle farming to game ranching in the Northern Cape Province’, South African Journal of Wildlife Research 37(1), 71–78.
http://dx.doi.org/10.3957/0379-4369-37.1.71 Commission of the European Communities (CEC), 2007, Implementing the new methodology for product market and sector monitoring: results of a first sector screening. Commission staff working document accompanying the communication on a single market for the 21st century. SEC (2007) 1517 final, 20 November 2007, viewed 20 July 2013, from
http://aei.pitt.edu/id/eprint/45896 Conningarth Economists, 2006, ‘Economic multipliers for the North-West Province’, unpublished research report, Pretoria. Cousins, J.A., Sadler, J.P. & Evans, J., 2010, ‘The challenge of regulating private wildlife ranches for conservation in South Africa’, Ecology and Society 15(2), Art 28, 21 pages. Development Bank of Southern Africa (DBSA), 2009, Social Accounting Matrices (SAMs), viewed 03 February 2013, from
http://www.dbsa.org/EN/DBSA-Operations/Proj/Tools/Pages/SAMS.aspx Dixon, P.B., Parmenter, B.R., Powell, A.A. & Wilcoxen, P.J., 1992, Notes and problems in applied general equilibrium economics, Volume 32, North-Holland, Amsterdam. Dorfman, R., 1993, ‘An introduction to benefit-cost analysis’, in R. Dorfman & N.S. Dorfman (eds.), Economics of the Environment: Selected Readings, 3rd ed., pp. 297–322, W.W. Norton, New York. Golan, E.H., Vogel, S.J., Frenzen, P.D. & Ralston, K.L., 2000, ‘Tracing the costs and benefits of improvements in food safety’, Agricultural Economic Report 791, Food and Rural Economics Division, Economic Research Service, US Department of Agriculture. Jafri, S.H.A. & Buland, D., 2006, ‘Economic impact of Erath County’s dairy industry’, The Texas Journal of Agriculture and Natural Resource 19, 8–22. Langholz, J.A. & Kerley, G.I.H., 2006, Combining conservation and development on private lands: An assessment of ecotourism-based private game reserves in the Eastern Cape, Report No. 56, Center for African Conservation Ecology, Nelson Mandela Metropolitan University. Lindsey, P.A., Roulet, P.A. & Romañach, S.S., 2007, ‘Economic and conservation significance of the trophy hunting industry in sub-Saharan Africa’, Biological Conservation 134(4), 455–469.
http://dx.doi.org/10.1016/j.biocon.2006.09.005 McDonald, S. & Kirsten, J.F., 1999, ‘South African agriculture and world market changes’, Agrekon 38(4), 535–547.
http://dx.doi.org/10.1080/03031853.1999.9524867 Musengezi, J., 2010, ‘Wildlife utilization on private land: Understanding the economics of game ranching in South Africa’, PhD thesis, Graduate School, University of Florida. PriceWaterhouse, 1994, The lowveld conservancies: new opportunities for productive and sustainable land use, Savé Valley, Bubiana and Chiredzi River Conservancies, Harare, Zimbabwe. Pyatt, G., 1988, ‘A SAM approach to modeling’, Journal of Policy Modeling 10(3), 327–352.
http://dx.doi.org/10.1016/0161-8938(88)90026-9 Rossouw, R. & Cloete, P.C., 2014, ‘Game ranching inter-sectoral linkages: A structural path analysis for South Africa’, Development Southern Africa 31(3), 373−396.
http://dx.doi.org/10.1080/0376835X.2014.887999 Round, J., 2003, ‘Social accounting matrices and SAM-based multiplier analysis’, in L.A.P. da Silva & F. Bourguignon (eds.), The impact of economic policies on poverty and income distribution: Evaluation techniques and tools (equity and development), pp. 301–324, World Bank Publications, Washington, DC. Ritchie, J.R.B. & Goeldner, C., 1994, Travel, tourism, and hospitality research, 2nd edn., Wiley & Sons, London. Sadoulet, E. & De Janvry, A., 1995, Quantitative development policy analysis, The John Hopkins University Press, Baltimore. Saayman, M., Van der Merwe, P. & Rossouw, R., 2011a, ‘The impact of hunting for biltong purposes on the SA economy’, Acta Commercii 11(1), 1–12. Saayman, M., Van der Merwe, P. & Rossouw, R., 2011b, ‘The economic impact of hunting in the Northern Cape Province’, South African Journal of Wildlife Research 41(1), 120–133.
http://dx.doi.org/10.3957/056.041.0115 Statistics South Africa (SSA), 2013, National and provincial labour market trends, 2003–2013. Statistical release P0211.4, Statistics South Africa, Pretoria. Taljaard, P.R., 2007, ‘The macro economy and irrigation agriculture in the Northern Cape Province of South Africa’, PhD thesis, Agricultural Economics, University of the Free State. Taylor, S., 2002, Save Valley conservancy [film], Zimbabwe, 60 minutes. Tisdell, C., 2006, ‘Valuation of tourism’s natural resources’, in L. Dwyer and P. Forsyth (eds.), International Handbook on the Economics of Tourism, pp. 359–378, Edward Elgar Publishing Limited, Cheltenham & Northampton, MA.
http://dx.doi.org/10.4337/9781847201638.00028 Tisdell, C., 2007, Economics, ecology and the environment. The economic importance of wildlife conservation on the Otago Peninsula – 20 years on, Working Paper No. 144, University of Queensland, Australia. Tisdell, C. & Wilson, C., 2004, ‘Economics of wildlife tourism’, in K. Higinbottom (ed.), Wildlife Tourism: Impacts, Management and Planning, pp. 451–468, Common Ground Publishing, Altona, Victoria. Townsend, R.F., 1997, Policy distortions and agricultural performance in the South African economy, Discussion Paper No. 138, Development Information Business Unit, Development Bank of Southern Africa, Pretoria, South Africa. Van der Merwe, P., Saayman, M. & Krugell, W., 2007, ‘The determinants of spending by biltong hunters’, South African Journal of Economic Management Sciences 10(2), 184–194. Weisbrod, G. & Weisbrod, B., 1997, Measuring the economic impact of projects and programs, Economic Development Research Group, Boston.
|